The current echocardiography practice mirrors the state of electrocardiography a decade ago. It remains a highly manual, strenuous and time-consuming process, requiring the expertise of trained specialists. Amid the growing burden of cardiovascular disease and strain placed on healthcare systems, current echocardiogram workflow limitations have led to workforce shortages,1 sonographer injuries,2 backlogs, heightened stress among healthcare professionals, and extended waiting times for patients requiring echocardiograms.3
The integration of artificial intelligence (AI) into cardiology represents a "Kodak moment" for those who might miss this transformative shift in medicine. Just as how photography company Kodak missed the digital photography revolution, overlooking Al's impact on cardiology could mean missing out on a seismic advancement in healthcare. AI is poised to reshape the way we diagnose, treat and manage heart conditions, offering unprecedented precision, speed and insights. Embracing AI in cardiology is not just about staying up to date, it is also about redefining the standards of care and ultimately saving more lives.
AI's transformative role in cardiology is evident in the realm of electrocardiography. Decades ago, ECG analysis was a highly manual process, requiring the measurement of electrical signal intervals with metal callipers and manual application of specific cut-offs or criteria to define abnormalities such as left ventricular hypertrophy. As a result, ECGs had to be read by specialists and were available only at cardiology centres. Today, AI integration has democratised ECG access, empowering not only GPs but also individuals keen on monitoring their cardiovascular well-being through smartwatches and other cutting-edge devices. How did this happen? The shift began with digital ECGs and early AI programs automating the interpretation of digital data by recognising fiducial points, making discrete measurements and defining common quantifiable abnormalities by predefined cut-offs. Advanced AI techniques, including deep-learning convolutional neural networks (CNNs), facilitate efficient, human-like ECG interpretation.4
Advancements and milestones
AI in medical imaging has the potential to establish an automated analysis pipeline for echocardiography, covering view identification, image segmentation, quantification of structure and function, and disease detection, thereby enhancing clinical diagnosis. In 2018, Madani et al demonstrated the potential of AI by using supervised deep learning with CNNs to automate echocardiogram view classification.5 Their CNN, trained on labelled still images and videos from 267 transthoracic echocardiograms, achieved an impressive 91.7% average accuracy, surpassing board-certified echocardiographies' 79.4% average accuracy.
Concurrently, Zhang et al used 14,035 echocardiograms gathered over a decade to train their CNN models.6 These models were trained to perform tasks including automatically identifying 23 echocardiogram views, segmenting cardiac chambers across five common views, quantifying chamber volumes and left ventricular mass, determining ejection fraction, and facilitating the automation of longitudinal strain detection via speckle tracking. Notably, automated measurements matched or surpassed manual measurements across eleven internal consistency metrics.
These concepts quickly moved into the clinical arena with a regulatory-cleared software product. In 2022, Tromp et al compared deep learning interpretation of 23 echo parameters by a commercially available AI software with assessments conducted by three core lab sonographers.7 Using the individual equivalence coefficient (IEC), which compares the disagreement between deep learning and human readers to inter-human disagreements, they found that among 602 anonymised echocardiography studies, IEC point estimates were all <0. This demonstrated that the disagreement between deep learning and human measures was lower than the disagreement among three core laboratory readers, highlighting AI's complete interchangeability with human assessors and its potential to introduce even greater consistency in evaluations compared to human experts. Additionally, AI analysis averaged just 1.2 minutes per echo study, compared to approximately 40 minutes for human core laboratory readers.
Transforming the heart failure management pathway
Long waiting times for echocardiography and the high demand for highly trained sonographers and cardiologists to examine heart disease patients remain a challenge. Enhancing access to diagnostic tools for early detection and treatment is necessary for timely intervention to prevent worsening conditions. Fortunately, combining point-of-care ultrasound (POCUS) with AI-automated echocardiography offers a solution. AI integration allows echocardiography procedures and interpretation to extend into primary care settings and even patients' home monitoring.
A point-of-care AI-assisted novice echocardiography for screening of heart failure (HF) study was conducted at the National Heart Centre Singapore.8 The study demonstrated the feasibility and efficacy of the AI novice pathway for HF screening. Novices who were trained for two weeks achieved a remarkable 96% yield in accurately acquiring 2D echocardiography images for HF screening. When combining AI-assisted echocardiography by novices with AI-automated image analysis, the AI-novice pathway outperformed N-terminal pro b-type natriuretic peptide (NT-proBNP) tests in detecting abnormal left ventricular ejection fraction (LVEF) <50%. The median time taken for novice laypersons to perform screening echocardiograms was only 11 minutes and 28 seconds.
A novice nurse-led screening programme for individuals at risk of chronic diseases in Tunisia further demonstrated the feasibility of using AI-POCUS as a task-shifting approach.9 The outcomes revealed that home-based AI-POCUS exhibited higher sensitivity than NT-proBNP in detecting LVEF <50% and left atrial volume index >34 mL/m2.
Findings from the groundbreaking OPERA study showed that AI interpretation of images from handheld echocardiography performed by nurses was comparable to the gold standards of HF diagnosis, which are cart-based echocardiography and interpretation by trained specialists.10 The enhanced clinical workflow reduced the echo wait times from approximately 12 months to less than six weeks at the National Health Service Greater Glasgow and Clyde in Scotland, UK.
Potential challenges and solutions
AI in echocardiography holds immense promise for revolutionising patient care and democratising access to this critical diagnostic tool. Some potential clinical applications of AI in echocardiography are shown in Figure 1. However, it also poses challenges.
Given AI's potential widespread impact on patient care, rigorous testing akin to medical device standards is important for algorithm development. Cloud-based solutions must ensure data privacy, security and regulatory compliance. Deep learning algorithms, sometimes viewed as "black boxes", can pose challenges in gaining clinician trust and understanding,11 necessitating transparency and interpretability for clinical acceptance and safety.
In conclusion, as the use of AI in cardiology advances, undergoes rigorous validation and is implemented responsibly, it is set to become an indispensable asset, benefitting both healthcare professionals and patients. Rather than replacing human experts, AI technology aims to empower them by streamlining manual tasks, allowing more time for meaningful patient interactions. The collaborative role of AI alongside human expertise will ultimately contribute to improved patient outcomes in healthcare's ever-evolving landscape.
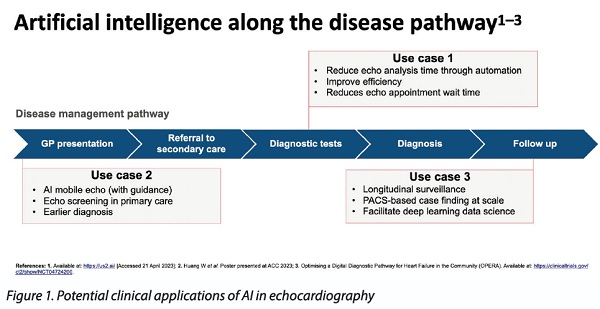